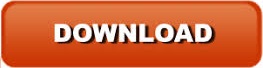
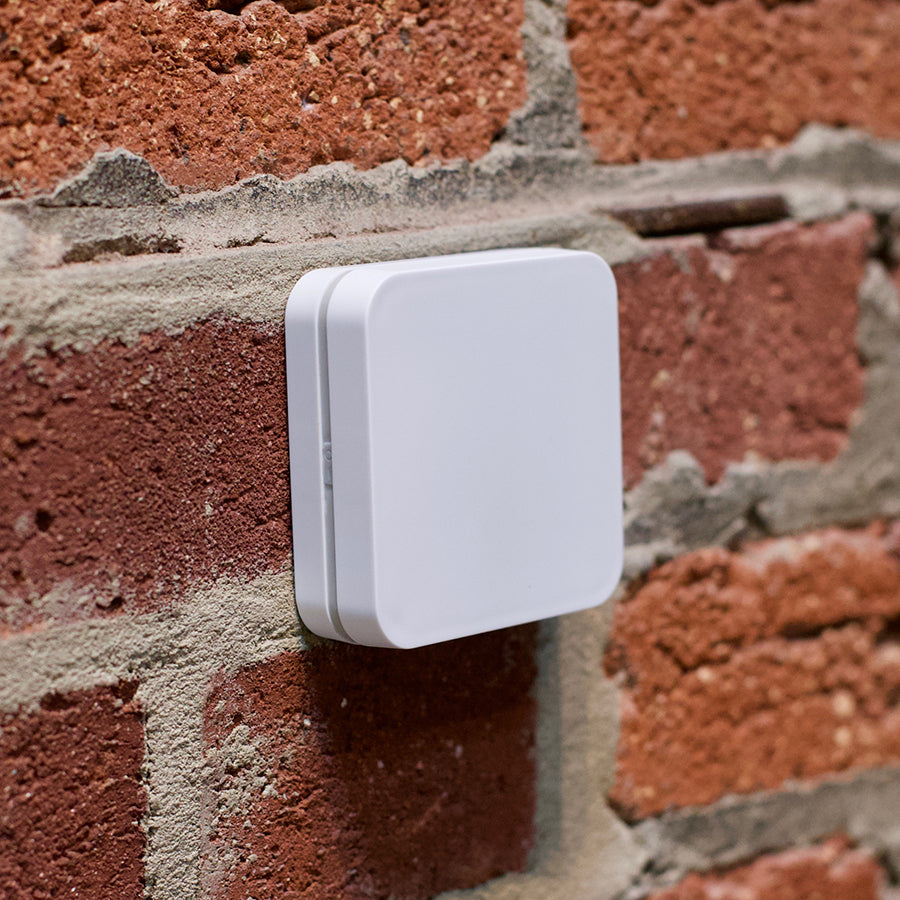
In indoor environments, lack of Global Positioning System (GPS) signals and line of sight with orbiting satellites makes navigation more challenging compared to outdoor environments. Current technological advancements enable users to encapsulate these systems in handheld devices, which effectively increases the popularity of navigation systems and the number of users. Navigation systems help users access unfamiliar environments. All of these techniques present a number of issues, including low precision, high computational complexity, and unreliability due to wireless channel impairments such as multipath effects caused by non line of sight (NLOS) propagation in indoor environments, while most positioning devices lack sufficient computing power.
Radiu network radbeacon dot Bluetooth#
Yet in the literature, in addition to the range-free techniques such as Centroid method and distance vector hop (DV-Hop) technique, typical ranging techniques based on channel state information (CSI), angle of arrival (AoA), time of arrival (ToA), time difference of arrival (TDoA), and radio signal strength indicator (RSSI) using various wireless technologies such as radio frequency identification (RFID), ultra-wide bandwidth (UWB), WiFi, and Bluetooth have been proposed for indoor positioning. All these transformational applications drive the need for accurate localization systems which require lots of resources due to the massive deployment of IoT devices.

The accuracy as a function of the history length (seconds) is displayed for the three classification methods: Thresholding with majority voting (Thresholding MV), Triangulation with majority voting (Triangulation MV), and the CNN+ANN network. The (†) symbol by the thresholding methods indicates that these methods only classified 18 zones, whereas the neural network methods classified 21 zones.Ĭlassification accuracy with history length. The method with the highest score for each metric is highlighted in bold. The scores for the precision, recall, F 1-score, and accuracy metrics are shown for all four classification methods.
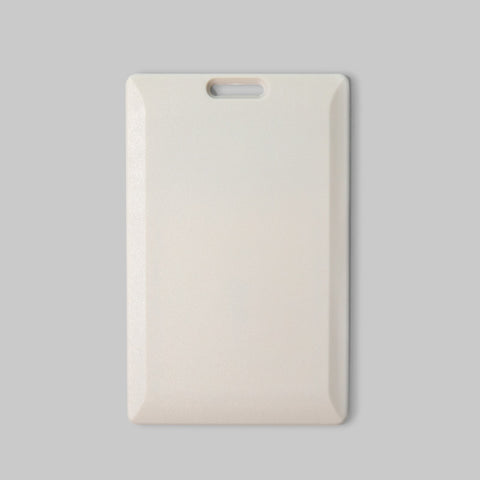
Precision, recall, and F 1-score results using the four classification methods (Thresholding, Thresholding with majority voting, CNN, and CNN+ANN) are shown. The categorical cross-entropy loss is shown for the convolutional neural network training as a function of the epoch number. Next, the resulting probability vectors are combined together and go through an artificial neural network, yielding the final classification.Ĭonvolutional neural network loss function. These images are then fed into the trained CNN shown in Fig 3. First, grayscale images are made for each second of data until the desired history length, indicated by T, is reached. The network structure for tracking the history of a tag is shown. Finally, a layer with the softmax activation function is used to obtain the classification. Then, the feature maps are flattened and input into a fully connected network. Initially, two convolution and max pooling layers are used.
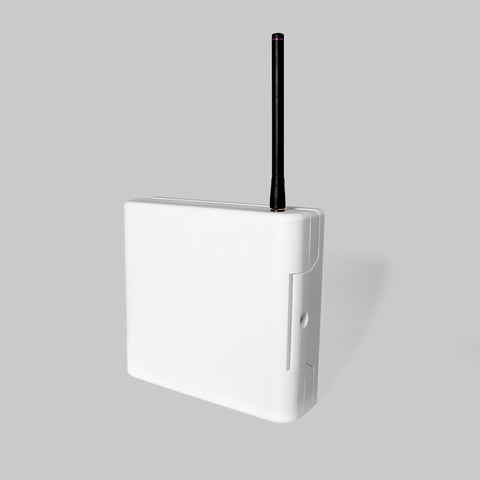
The structure of the convolutional neural network is displayed. Two RadBeacon Dot (Radius Networks) tags were tested in two different exam rooms, and both show decreasing signal strength as the tags are placed further from the Raspberry Pis.Ĭonvolutional neural network architecture. The relative received signal strength (RSSI) is shown as a function of distance from the node. Signal intensity as a function of distance from node. White represents a high RSSI value and black represents a low RSSI value in the images. In the grayscale images, each pixel with signal corresponds to a Raspberry Pi node, and therefore there are only 20 elements in the 34 x 15 image that vary signal as a function of time and tag position. A) A schematic of the floor plan for the first floor exam area where the experiments were conducted B) A representative grayscale image used for training when the tag is in exam room 1.2, and C) A representative grayscale image when the tag is in exam room 1.8.
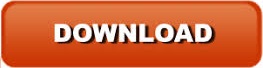